A guide to forecasting at Founders Pledge
A practical guide to forecasting, guidelines for better forecasting, and how to harness it to improve our work.
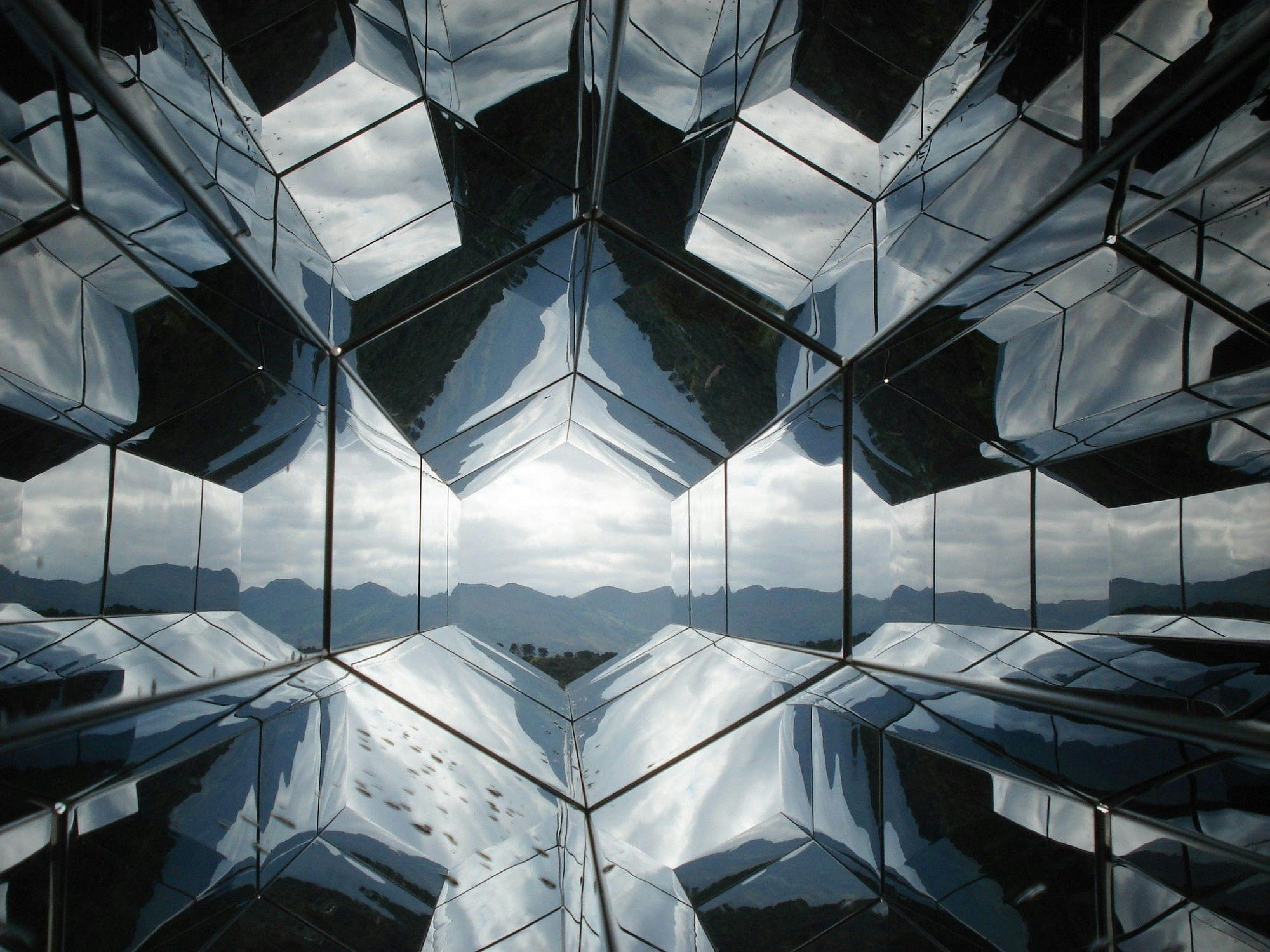
▲ Photo by drmakete lab on Unsplash
Related research
This is an introduction to our guide to forecasting at Founders Pledge.
Introduction: Follow the Data
At Founders Pledge, one of our core values is “follow data.” This means not only that we rigorously evaluate evidence, but also that we want to understand where the data we use has been and where it is going. Where will the data be a year, a decade, or even a century from now? In short, we want to understand the future as well as we can, and what our members can do to make that future better than the present. Much of our work therefore is fundamentally an exercise in forecasting.
Because we rely so fundamentally on forecasts — even when we don’t recognize them as such — we want to follow evidence-based practices to make these predictions more accurate. This document outlines a variety of such practices and how we can apply them in our research process and integrate them into the research we produce.
When done correctly, these practices can be powerful tools in our research toolkit. They can make our evaluations more accurate, streamline research, and automate processes. More importantly, forecasting is a learnable skill. Research on the traits shared by the most elite forecasters — known as “superforecasters” — suggests that they engage in “patterns of behavior suggestive of the view that forecasting skill can be cultivated via deep deliberative practice.”1
The following pages are a practical guide to this “deep deliberative practice” of forecasting, and how to harness it to improve our work.
What Is Crowdsourced Forecasting?
In this section, I explain crowdsourced probabilistic forecasting and summarize the evidence behind it. In short, experiments conducted by independent researchers and initiatives of the U.S. intelligence community show that crowdsourced forecasts can outperform subject-matter experts and provide a reliable tool to understand the future. At the moment, these tools are confined to well-defined, narrow, short-term problems, but there are ongoing attempts to leverage forecasting for longer-term and “big question” problems. In the next section, I explain how these tools could help support the work of the research team and “reinforce the foundations” at Founders Pledge.
Definitions and Background
Probabilistic forecasting refers to the practice of assigning numerical probabilities (or probability ranges) to falsifiable future events, and then scoring and evaluating performance. This practice is familiar from weather forecasting, and widespread in medicine, finance, and even sports betting. It is also becoming more common on geopolitical and social issues, especially in the world’s intelligence communities.
Crowdsourced probabilistic forecasting refers to the practice of aggregating a collection of such forecasts to take advantage of the “wisdom of the crowds.” Such aggregation can occur either on prediction markets or forecast aggregation platforms2. Prediction markets use money — national currencies, “play” money, tokens, or cryptocurrencies — to trade on information (often in the form of binary yes-or-no options) such that the price of the prediction contract can reflect the crowd’s estimate of the probability of an event. Examples of active prediction markets include PredictIt and Polymarket.
Forecast aggregation platforms combine individual forecasts algorithmically without a market. Examples of active forecast aggregation platforms include Good Judgment Open and Metaculus. Each type of platform has its advantages and disadvantages, but behavioral science experiments suggest that aggregation algorithms can be as accurate as markets, and outperform markets under certain conditions.3
Brier Scores are a common measure to score and track forecaster accuracy. Superforecasters is a term used to describe the most high-performing group of forecasters, developed to describe the winning strategy of a U.S. intelligence community forecasting tournament.4
Behavioral science researchers over the past two decades — often working together with the U.S. intelligence community — have shown that the “wisdom of the crowd” can outperform individual experts and have uncovered tools for increasing the accuracy of forecasters and teams of forecasters. For example, using certain statistical methods for aggregating forecasts can help to elicit collective intelligence; an improved aggregation algorithm helped the Good Judgment Project win a forecasting tournament and attain 35% improved accuracy when compared with the unweighted forecast average.5
The continued effort to optimize aggregation algorithms has paid off. For example, a 2021 analysis of disease outbreak forecasting (funded by Open Philanthropy) found that 0 of 562 forecasters outperformed their aggregate crowd forecast on 61 forecasts.6 In other words, the optimally-aggregated wisdom of the crowd was higher than the forecast of any one individual member of that crowd.
Despite its strengths, however, crowdsourced forecasting is no panacea. Evidence is especially limited for the accuracy of long-term forecasts over 10 years.7 Moreover, to rigorously score forecasts, forecast questions must be falsifiable and highly specific and often focus on “narrow” problems, whereas decision-makers may wish to know the answers to broad questions about the “big picture”, such as “Will U.S.-China competition intensify over the next 5 years?”8 To address this issue, Philip Tetlock and others have suggested combining scenario planning with probabilistic forecasting, an approach recently put into practice with the Center for Security and Emerging Technology’s tech-forecasting platform, CSET Foretell, now known as INFER.9 Foretell/INFER uses forecasts on specific near-term predictors and metrics to inform “trend departures” from large-scale scenarios.10 For example, CSET used forecasts on the volume U.S.-China trade, U.S. visas issued to Chinese nationals, on public opinion of China, and on Chinese incursions into allied air space to forecast “increasing U.S.-China tensions.”11 In short, there are models for how to overcome the shortcomings of crowdsourced forecasts and incorporate them into decision-relevant research questions, though they have not been tested extensively.
Continue reading to learn more about the forecasting process, how to become a better forecaster, and how we use it at Founders Pledge.
Notes
-
Barbara Mellers et al., “Identifying and Cultivating Superforecasters as a Method of Improving Probabilistic Predictions,” Perspectives on Psychological Science 10, no. 3 (May 1, 2015): 277. ↩
-
For comparisons of prediction markets and non-market aggregation platforms see Pavel Atanasov et al., “Distilling the Wisdom of Crowds: Prediction Markets vs. Prediction Polls,” Management Science 63, no. 3 (March 2017): 691–706. ↩
-
Ibid. and Jason Dana et al., “Are Markets More Accurate than Polls? The Surprising Informational Value of ‘Just Asking,’” Judgment and Decision Making; ↩
-
Barbara Mellers et al., “Identifying and Cultivating Superforecasters as a Method of Improving Probabilistic Predictions,” Perspectives on Psychological Science 10, no. 3 (May 1, 2015): 267–81. ↩
-
Philip E. Tetlock et al., “Forecasting Tournaments: Tools for Increasing Transparency and Improving the Quality of Debate,” Current Directions in Psychological Science 23, no. 4 (August 1, 2014): 291. ↩
-
Tara Kirk Sell et al., “Using Prediction Polling to Harness Collective Intelligence for Disease Forecasting,” BMC Public Health 21, no. 1 (December 2021): 2132. ↩
-
For an overview of this issue, see “How Feasible Is Long-Range Forecasting?,” Open Philanthropy, October 10, 2019. ↩
-
“Admittedly, the specificity required to make questions rigorously resolvable precludes asking “big” questions” Philip E. Tetlock, Barbara A. Mellers, and J. Peter Scoblic, “Bringing Probability Judgments into Policy Debates via Forecasting Tournaments,” Science 355, no. 6324 (February 3, 2017): 482. ↩
-
Center for Security and Emerging Technology, “Future Indices: How Crowd Forecasting Can Inform the Big Picture” (Center for Security and Emerging Technology, October 2020): 16, footnote 4. (CSET Foretell is now a part of the Applied Research Laboratory for Intelligence and Security, and known as INFER) ↩
-
For an overview of this method, see Center for Security and Emerging Technology, “Future Indices: How Crowd Forecasting Can Inform the Big Picture” (Center for Security and Emerging Technology, October 2020). ↩
-
Ibid., 7. ↩