Replicability and Generalisability: how do experimental effects translate to the real world?
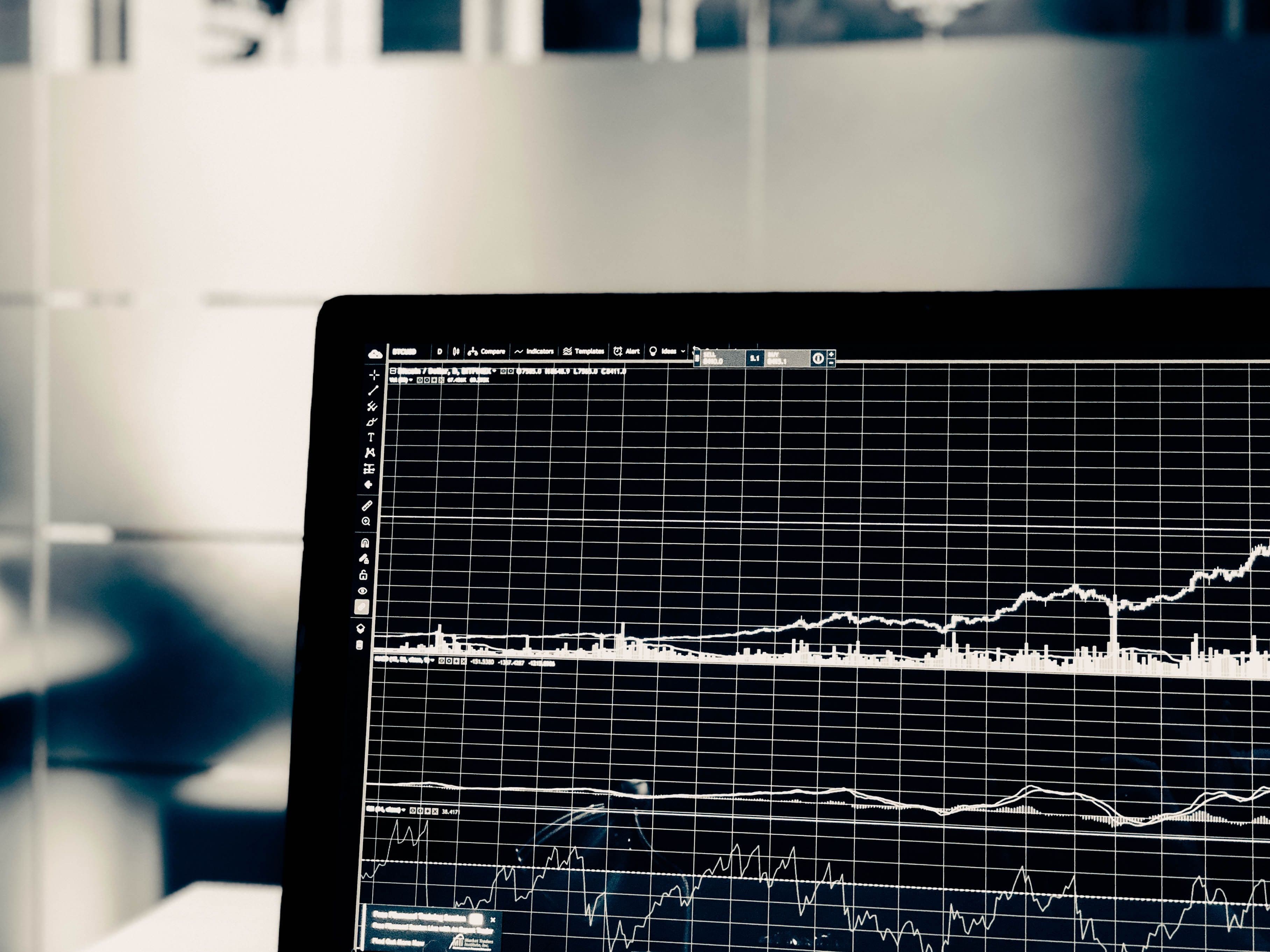
▲ Photo by Chris Liverani on Unsplash
This is a summary of our guide to cost-effectiveness analyses discounts
Summary
Experimental measures of effect sizes (how well an intervention will work) do not always predict the real-world effects of interventions with high accuracy. In this report, we review evidence from the empirical literature to better understand how experimental effects tend to translate into the real-world. We suggest that researchers seeking to predict the effects of real-world interventions should attend to two factors; the internal reliability of a study (would the researchers find the same effect, if they ran exactly the same study again?) and the external validity of a study (does the result generalise to different contexts, beyond the specific context that the intervention was experimentally tested within?) We provide a systematic framework for researchers seeking to estimate the impact of these two factors, that takes into account components such as publication bias, methodological error, statistical power, and contextual differences between the study population and the new population that stands to benefit from the intervention. This framework aims to improve our ability to predict how well new interventions will work in different areas, informing our cost-effectiveness analyses.
Introduction
At Founders Pledge, we use cost-effectiveness analyses to predict the impact of particular interventions or charities per amount of money that is donated. This entails estimating the likely impact of a given intervention. For example, how strongly does an anti-malarial net affect mortality, or a deworming pill affect future income? Within our analyses of interventions related to global health and development, our estimates of these impacts usually come from randomised controlled trials (RCTs) or quasi-experimental studies. However, these effect sizes might not accurately represent the true effect that we would actually observe from a particular intervention. For example, effect sizes reported in an RCT may be inflated due to bias or a lack of statistical power. In addition, effect sizes that were found in one context might not be representative of the effects that we should expect in a new context. To account for these issues, we usually apply discounts to RCT/ quasi-experimental results within our cost-effectiveness analyses. The purpose of this work is to assess the degree to which these results replicate and generalize, in order to better standardise our processes for estimating these discounts.
Replicability and generalisability are easy to conflate. Replicability (in the context in which we use it)1 refers to whether an RCT would replicate, if it were to be rerun; to what extent does the study provide strong evidence for its findings? Generalisability refers to whether RCT results will generalise to new contexts; will the same intervention work, in a new area? It is possible that an RCT with excellent replicability will have poor generalisability. I have therefore split up these discounts into two components; internal reliability (corresponding to replicability) and external validity (referring to generalisability; see Fig 1). Since these two aspects of validity have different causes, I suggest that we should structure our discounts in this manner— this also matches GiveWell’s approach.
Following Gelman and Carlin (2014), I split internal reliability itself into two components. First is the Type M error, or whether the magnitude of the effect size is correct. Second is the Type S error, or the probability that the study’s measured effect is in the wrong direction. I chose this distinction because it does not rely upon p-values, as we move towards increasingly Bayesian methods (for criticism of p-values, see (Burnham & Anderson, 2014; Ellison, 2004; Gardner & Altman, 1986). At the same time, this approach is generally compatible with existing literature which uses null hypothesis testing and p-values. In the case of Type M errors, I argue that we can estimate effect size inflation by examining statistical power and likelihood of bias. I argue that Type S errors are likely to be fairly uncommon for the type of studies that we typically work with, but will need to be accounted for in certain situations.2 Overall, I estimate that the median effect size (that we might use in our CEAs) is likely to require an internal reliability Type M adjustment of ~50-60%.3 However, I highlight that there is also a lot of variation here.
A theme that underlies this internal reliability work—and that I suspect has been previously underappreciated—is the importance of statistical power, and its interaction with publication bias in generating inflated effect sizes. Power is the ability of a statistical test to correctly detect an effect, assuming that it is there. While low power is well known to increase the rates of false negatives, it is less well-appreciated that low power also increases effect size inflation (when conditioned upon statistical significance; Button et al., 2013; Gelman and Carlin 2014). This is because underpowered studies will tend to only successfully identify that an effect is present when the effect size is inflated, for example due to random error (see Question 2 for full explanation). My best understanding is that this effect underlies a large amount of replicability variation across experimental work.4 The upside to this is that we can get quite far (in determining the likely inflation of an effect size) by attending to study power. The downside is that it is often difficult to calculate a study’s power—it is possible that the approach outlined here will prove impractical—although I have created some guidelines and rules-of-thumb below.
A second theme that underlies this work is the importance of forming baseline estimates of the likely effect of an intervention, independent of the study at hand. We should be somewhat skeptical of ‘surprising’ results—interventions that appear to work well (according to the effect size), but their mechanism is poorly understood. Perhaps unsurprisingly, the rate of false positives in the experimental literature is higher when researchers investigate hypotheses with lower prior odds of being true (Ioannidis, 2005). In line with the idea that forming baseline estimates is important, note that while replication studies have frequently indicated that studies frequently fail to replicate (e.g. Camerer et al., 2016; Open Science Collaboration, 2015), people do appear to be reasonably good at predicting which studies will replicate. For example, Forsell et al. (2019) found that prediction markets correctly predicted 75% of replication outcomes among 24 psychology replications—although people were less willing to make predictions about effect sizes. As these efforts proceed, I think it will be possible in the future to use people’s predictions to form our baseline estimates of whether given interventions tend to work (e.g. on the Social Science Prediction Platform).
External validity refers to the extent to which an RCT/ quasi-experimental result will generalise, if the same study is undertaken in a different context. Although I view external validity as being probably more critical than internal reliability in determining an intervention’s success,5 there is a far smaller amount of relevant evidence here. I take the view that we should approach this question mechanistically, by examining (1) the extent to which the conditions required for the intervention to work hold in the new context (note that we should expect some RCTs will have effects going in the opposite direction when tested in a new context); (2) whether the effect in the RCT was larger due to participants realising that they were being watched (social desirability biases); (3) whether there are emergent effects from the intervention that will appear once it is scaled up, and; (4) whether the intervention will be conducted in a different way (i.e. less rigorously) when completed at scale (see; Duflo & Banerjee, 2017). Existing empirical work can ground our estimates. For example, Vivalt’s (2020) work suggests that the median absolute amount by which a predicted effect size differs from the same value given in a different study is approximately 99%. In addition, I have created a library of our current discounts here so that researchers can compare their discounts relative to others.
As a note, I have only briefly considered quasi-experimental evidence within this work, and have generally focused upon RCTs. Many of the points covered in this write-up will also apply to quasi-experimental work, but researchers assessing quasi-experimental studies will need to spend longer on assessing methodological bias relative to the guidelines suggested here for an RCT (e.g. establishing causation; see here).
Overall, this work aims to create clearer frameworks for estimating the appropriate discounts for RCTs. I think that this work is unlikely to affect well-studied interventions (which have high power anyway), and is likely to be most helpful for establishing discounts for ‘risky’ interventions that are comparatively less well-studied.
Notes
-
I am using ‘replicability’ to refer to exact replications, where replications occur with the same study population and same study methods. In the broader literature, ‘replicability’ is sometimes also used to refer to conceptual replications, where the key finding is replicated even if the context and experimental methods are different. ↩
-
Namely, when the study is low-powered, the data is noisy, and it is mechanistically possible that the effect could go in the other direction. For example, this seems plausible for some interventions that work by shifting behavior. ↩
-
That is, multiplying the study’s reported effect size by 0.5-0.6 will generate our best estimate of the true effect size. Note that Founders Pledge evaluates a number of interventions were there is a smaller evidence base than say, anti-malarial bednets where there are a large number of studies. ↩
-
Given that power seems to be a key underlying cause, I have focused more on examining this root cause rather than creating universal lists of deflators. Note that power differs across academic fields and sub-fields, according to factors such as the expected effect size and norms around sample sizes. However, universal deflators for components that are unrelated (or at least, mostly unrelated) to power may be helpful; see Fanelli (2017) for some estimates here. ↩
-
As in, I would predict that a larger degree of the variation in FP/ GiveWell’s recommended charities’ success probably stems from external validity rather than internal reliability. ↩