How we use Elo ratings to evaluate long-term funding opportunities
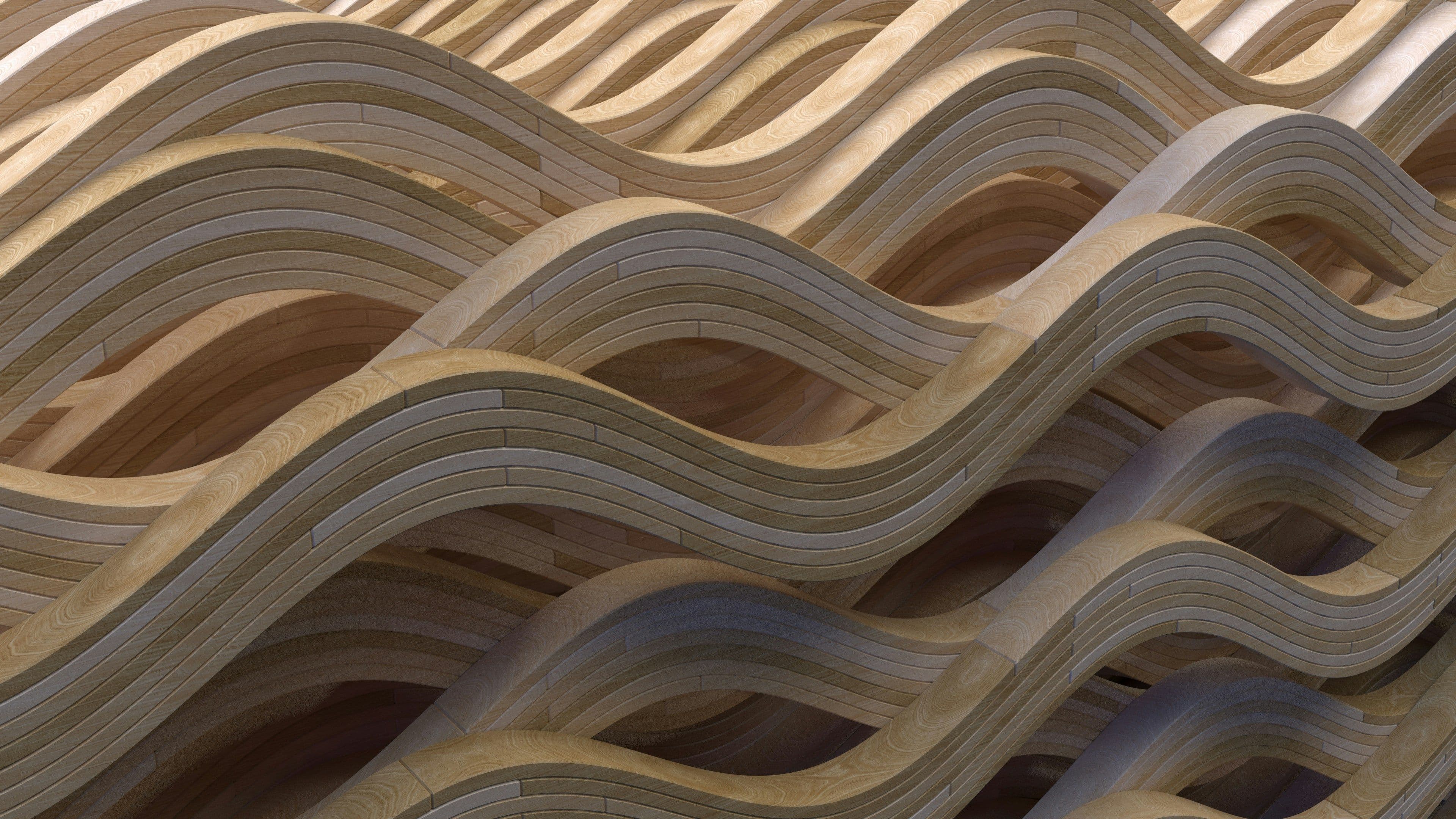
▲ Photo by Getty Images for Unsplash
When we evaluate certain funding opportunities (FOs), we face a fundamental challenge: How can we quantify the impact of interventions in high-uncertainty contexts that are poorly-suited to absolute cost-effectiveness analyses (CEA)? In the absence of meaningful empirical data or direct feedback loops, attempts to quantify impact can feel like pulling numbers out of thin air.
Developing a rigorous framework for evaluating relative impact in high-uncertainty contexts, such as climate change and global catastrophic risks, has been a major focus of our research team’s work. We’ve published cause-area-specific research reports and notes about our methodologies, including:
- How we evaluate relative impact in high uncertainty contexts.
- The most important heuristics we believe philanthropists can use to identify high-impact interventions related to advanced AI.
- The most important heuristics we believe philanthropists can use to identify high-impact interventions related to biological risks.
- The introduction of right-of-boom interventions and how philanthropists can approach the search for “the nuclear equivalent of mosquito nets.”
- Why the most fashionable climate interventions aren’t necessarily the most effective ones.
As part of these efforts, our research team recently developed a new charity ratings system based on pairwise comparisons between funding opportunities, inspired by the Elo ratings system used in chess. This methodology allows charity evaluators to convert qualitative reasoning into quantitative scores in a rigorous way.
This Elo-style ratings system is especially relevant when we evaluate “long-term” FOs that affect the long-term future, which will be our primary focus in this post. However, it’s worth noting that this methodology can also be broadly applied to the goal of quantifying relative impact in any high-uncertainty context.
This isn’t a perfect system, but it’s a step closer to a ratings system that’s appropriate for the context of charity evaluation. We hope that this post can help guide foundations, charity evaluators, and individual philanthropists facing similar methodological challenges.
This post will:
- Outline the challenges with quantifying long-term FOs.
- Explain our Elo-style ratings system and its key advantages.
- Describe the evolution of this methodology.
- List open questions we’re considering as we move forward.
The challenge of quantifying long-term funding opportunities
Our mission at Founders Pledge is to do the most good possible with the resources available. There are numerous charities we could recommend to our members, but only so much funding to go around.
Just like other charities, long-term charities differ widely in cost-effectiveness. An additional donation to one high-impact funding organization (HIFO) could save ten times as many future lives as the same donation to a different organization. It’s important to make sure we choose high-impact FOs, so we can help our members maximize the effectiveness of their donations.
When we think and talk about maximizing impact, simply using qualitative terms can easily lead to miscommunication. Studies have found that people can interpret the phrase “a real possibility” as anything from a 20% probability to an 80% probability.1 Similarly, saying that a longtermist FO is “very impactful” isn’t sufficient for recommending it to our members, or for distinguishing it from other FOs. Using rigorous numbers is crucial for ensuring that we’re all on the same page about which FOs are most impactful and why.
How do we typically quantify impact?
When we evaluate FOs in global health and development, we have a well-developed toolkit for estimating how much impact a certain donation will make. Our Global Health and Development team shares a CEA-based methodology with other charity evaluators like GiveWell. Among other methods, we:
- Use data from randomized controlled trials, where we can see the effect of a certain intervention compared to the counterfactual of what the outcome would have been without that intervention.
- Cite impact evaluation data from similar charities, interventions, and organizations.
- Check the outcomes of predictions we’ve made in the past to help calibrate our future predictions.
These methods are often able to estimate expected absolute impact in terms of metrics such as lives saved per dollar spent. Knowing absolute impact makes it trivial to figure out relative impact—which FO we believe to be most impactful out of all the FOs we could choose to fund.
What makes long-term impact difficult to quantify?
Compared to FOs in global health and development, it’s much more difficult to estimate absolute impact for long-term FOs. This is true for multiple reasons:
- There are fewer visible feedback loops between what we fund and what the outcomes will be, so many of our usual tools, like randomized controlled trials, don’t easily apply.
- Some longtermists view the value of the future as astronomically large (and hence the cost of an existential catastrophe as astronomically large). This makes it easy to subjectively justify any intervention with even an extremely low probability of success. This opens up a lot of room for motivated reasoning and other biases.
- Many long-term FOs are inherently “hits based,” meaning a very small number of highly successful FOs account for most of our impact, with a much larger number of unsuccessful FOs. Thus, we may not have good base rate estimates of their probabilities of success, since the best FOs are high-risk.
We can sometimes attempt to quantify interventions using CEA-like approaches, Monte Carlo simulations, and other tools designed for similar problems. For example, our nuclear report contains an illustrative spreadsheet on how nuclear risk-reduction cashes out in current-generations terms. Other researchers, like Matheny (2007), Millett and Snyder-Beattie (2017), and Schulman and Thornley (2024), have taken a similar “common sense” approach to cost-effectiveness for existential risk. In theory, such approaches could also draw on the results of aggregated estimates from probabilistic forecasting platforms and tournaments.
Many such approaches, however, are not well-suited for (or are difficult to operationalize in) the context of high-throughput charity evaluation, where charity evaluators have to rapidly rate many potential grants when the opportunity costs are high. These approaches may also suffer from various pitfalls, which we aim to avoid in our charity evaluation methodologies:
- We aim to avoid subjectivity: relying on expert estimates that aren’t necessarily grounded in evidence.
- We aim to avoid spurious rigor: a quantitative system that appears rigorous on the surface, but in which numbers come from thin air.
- We aim to avoid bias toward the quantifiable: prioritizing interventions that are easy to put into a spreadsheet, as opposed to ones that might be high-impact but for which we have fewer available numbers.
- We aim to avoid overoptimization: letting the perfect be the enemy of the good, e.g., trying to come up with a system that satisfies all possible goals.
Our Elo-style ratings system
The question of how to quantify something that’s difficult to quantify arises in many fields. One such field is chess.
In chess, each player needs a way to accurately communicate their strength as a player. This has historically been difficult to do, since chess is a complex game that requires multiple types of strategies and skills. To solve this, the World Chess Federation uses the Elo ratings system, which gives each player a single rating. Elo ratings are constructed and continuously refined based on the outcomes of games, using the following principles:
- All unrated players begin with the same number of points.
- Every time two players finish a rated game, the winner “takes” points from the loser.
- The number of points gained or lost depends on the initial points differential between the two players, such that an upset win leads to a higher transfer of points than a win between two players with similar ratings, which ensures that this system is self-correcting.
How do we apply Elo ratings to long-term evaluations?
The Elo ratings system works well for the challenge of evaluating long-term interventions. This is because even in cases where we lack the necessary data to determine the absolute impact of any individual intervention, we often do have enough evidence to determine the relative impact between that intervention and another one.
Just as chess ratings are constructed using head-to-head games between pairs of players, we can construct our own ratings based on head-to-head comparisons between pairs of funding opportunities. In these comparisons, the only thing we need to do to derive a rating is determine a dominance relation: Is Charity A “better” than Charity B?
Each matchup can lead to one of three outcomes: Charity A winning, Charity B winning, or a tie between the two.2 Over the course of multiple matchups between different pairs of FOs, we can derive a reasonably accurate rating for each of them and decide which ones should be considered HIFO.
Key to the success of this approach are two requirements:
- Dominance relations must be explained and defended. Researchers rating an existing or candidate HIFO must be able to explain why “matchups”—comparisons between a pair of programs—shake out the way they do. These outcomes must be evidence-based and qualitatively rigorous. To decide these outcomes, we look at heuristics we call impact multipliers: features of an impact landscape that make some FOs more effective than others. We’ll discuss impact factors in more depth in the section below.
- Each funding opportunity must go through multiple comparisons. By default, a candidate HIFO begins with a rating of 1000 (untransformed). In order to be rated, it need only be compared with a single existing HIFO. But the premise of our system is that ratings approximate the “true” underlying value of a HIFO over time. In order for that to be true, we need to make multiple comparisons.
How do we decide the outcome of each matchup?
To determine the outcome of each matchup in our Elo ratings system, we look at the impact multipliers3 we’ve identified that distinguish higher- from lower-impact funding opportunities within a given cause area. FOs that stack impact multipliers, creating a “conjunction of multipliers,” are dramatically more effective than the average FO.
A significant part of our research process focuses on understanding how each cause area is structured so we can pinpoint its most important impact multipliers. We share our findings for each cause area in our in-depth investigations and reports, such as those on AI, climate change, air pollution, and biological risks.
As a simplified example, let’s say we’re trying to evaluate a hypothetical organization called BioPSA, a nonprofit that researches catastrophic biological risks and briefs the U.S. government on those risks. We’d like to compare BioPSA to another hypothetical organization called VaccDev, a research lab that works on vaccine development for potentially catastrophic pathogens.
Both BioPSA and VaccDev do important work to address long-term biosecurity risks. Based on our research team’s investigations, these are some of the impact multipliers we would consider in that cause area, all else being equal:
- An FO that prioritizes robustness to extreme threats (i.e., catastrophic and existential pandemics) is more likely to be high-impact than an FO that focuses on responding to moderate disease outbreaks.
- An FO that focuses on pathogen- and threat-agnostic approaches is more likely to be high-impact than an FO that focuses on a specific pathogen or threat.
- An FO that leverages existing society resources using advocacy-based interventions is more likely to be high-impact than an FO that relies on in-house resources.
To determine whether BioPSA or VaccDev is more likely to be high-impact, we can consider the following factors:
- Both BioPSA and VaccDev prioritize robustness to extreme threats by addressing potentially catastrophic risks.
- However, BioPSA takes a more threat-agnostic approach than VaccDev, focusing on all future biosecurity risks rather than pathogen-specific ones.
- Furthermore, by influencing government resource allocation, BioPSA leverages existing societal resources, while VaccDev only focuses on in-house projects.
So, in this matchup, all else being equal, we would determine that BioPSA “wins” over VaccDev.
How are ratings calculated?
At the calculation level, our long-term ratings are determined almost exactly as Elo ratings are, with new FOs entering with an assumed score of 1000 and the calculation using a “k-factor” of 324, where the k-factor is the maximum possible adjustment after a single game. The k-factor determines how quickly an FO’s rating updates, so choosing the optimal k-factor is important for ensuring that we account for new matchups without overreacting to them.5
In order to express order-of-magnitude impact differentials between these FOs, we transform our Elo-style ratings such that a 200-point difference in untransformed ratings works out to an order-of-magnitude difference in final ratings. Thus, an FO rated 1000 (untransformed) has a rating of 100 (transformed), an FO rated 1400 (untransformed) has a rating of 10,000 (transformed), and an FO rated 800 (untransformed) has a rating of 10 (transformed).
Let’s return to our hypothetical example with BioPSA. The following table summarizes comparisons between BioPSA and four hypothetical FOs we would make using the impact multipliers our research team has identified for biological risks, alongside the resulting changes to their ratings:
A | B | Winner | New A Elo | New B Elo |
---|---|---|---|---|
BioPSA | VaccDev | BioPSA | 130 (+30) | 462 (-141) |
BioPSA | NewBio | NewBio | 110 (-20) | 204 (+32) |
BioPSA | RiskGo | BioPSA | 130 (+20) | 72 (-14) |
BioPSA | AITeam | BioPSA | 160 (+30) | 155 (-46) |
After these four matchups, BioPSA would emerge with a rating of 160. Using this rating, we can gain clarity into how BioPSA compares to our existing FOs and determine whether or not it should be considered HIFO.
Because our own internal Elo model contains confidential information, we are unable to share it publicly. If you work in charity evaluation and are interested in a more in-depth explanation of how to construct a simple spreadsheet-based model for Elo ratings, you can reach out to us at research@founderspledge.com.
What are the advantages of this system?
This system has a number of valuable qualities.
- It is conceptually sound. Under certain assumptions, across multiple pairwise comparisons, untransformed ratings accurately represent cardinal relationships between FOs.
- It is Bayesian. The Elo system allows updating of ratings based on the strength of evidence (with more evidence offered, for instance, by a new FO “beating” a highly-rated existing HIFO).6
- It communicates large impact differentials. Transformed ratings allow the cardinal relationships to be re-represented in a format that communicates to stakeholders the dramatic impact differentials that we expect to exist across long-term opportunities.
- It is efficient. Unlike more complex systems requiring more extensive deliberative or mathematical processes, our system requires only (at minimum) a single pairwise comparison between an FO candidate and a comparator.
- It is easy to understand. In contrast to systems that rely on complicated causal models or voting systems, ours is grounded in an easily explicable, well-tested system, with a procedure that is trivial to understand.
- It aggregates information effectively. The Elo system can elicit truths about the world that may not be explicit in any individual rating or to any individual evaluator; like the “wisdom of the crowd,” this system harnesses collective intelligence that may otherwise go unnoticed.
- It is flexible and easy to update. The Elo approach allows us to remain flexible in evaluating opportunities, and can encompass a range of rigor, from fast grants to deep impact-multiplier-based evaluations.
The evolution of our long-term evaluation system
In the past, our system for evaluating long-term FOs relied on a concept called “Petrov7 points,” where one Petrov point represents a one-hundred-millionth of a percent improvement in the value of the whole future per million dollars.
To evaluate an FO, members of our research team would convene to determine how many Petrov points we expected that FO to be worth. We defined “improvement in the value of the whole future” as a function of two variables:
- The reduction in the chance of an existential catastrophe this century.
- The improvement in the value of the future, conditional on there being no existential catastrophe this century, e.g., how many people there are, how their lives are improved, and the reduction in the risk of existential catastrophe beyond this century.
Conceptually, it makes sense to think about long-term cost-effectiveness this way, and several other charity evaluators use similar systems for evaluating long-term FOs. For example, Rethink Priorities uses basis points for billion (bp/bn), which estimates risk reduced per billion dollars spent.
What are the drawbacks of the Petrov points system?
The Petrov points system had the following weaknesses:
- It was complicated to implement, requiring a deliberative process incorporating large numbers of team members for an extended period of time.
- It was irreducibly subjective, in that estimates by team members were not fundamentally grounded in empirics.
- It featured units (“Petrov points”) that were difficult to explain to outside stakeholders, in that their relationship to real-world quantities (“risk of extinction”) was both unclear and hard to justify.
- It was extremely time-intensive to update and maintain. Every time we refreshed our evaluation of an FO, we needed to re-convene and hold a new discussion. This came with a high opportunity cost, as our researchers could have spent that time finding new HIFO and generating more impact.
Fundamentally, this system was both complex to implement and difficult to communicate, making it in some sense the worst of both worlds.
How did we come up with the Elo approach?
For several years, we considered different approaches to replace the Petrov points system. Initially, we tried to use dimensionless, quantitative impact multipliers to derive ratings, but quickly discovered that the implementation of such a system was rife with uncertainties and too complex to be dependable.
In 2023, one of our researchers, Tom Barnes, suggested applying Elo ratings to this problem. To test out this approach, the research team derived initial ratings as a group by conducting pairwise comparisons between existing HIFO. These initial ratings, which were necessary to get the system started, ultimately matter less and less each time we update them with new information.
Open questions
Our Elo-style ratings methodology is still relatively new, with room for improvement. We’re considering several open questions as we iterate on our long-term ratings system going forward:
- What’s the ideal number of minimum comparisons? With more comparisons, we expect to get a more accurate understanding of each FO’s impact.
- Should the outcome of each matchup be treated as a binary outcome or as a probability? In chess, the winner of a game is established without any doubt, but that’s rarely the case with comparisons between long-term FOs. To capture that, we could potentially update our ratings system to reflect probabilities, e.g., “We’re 90% sure that Charity A wins over Charity B, with a 10% chance that Charity B wins over Charity A.”
- Should more recent ratings be weighted more heavily? The philanthropy landscape changes over time, and there’s a case to be made that we should in theory prioritize the outcomes of more recent matchups over older ones.
- How can Elo ratings be integrated with other tools, such as Monte Carlo simulation-based methods for quantifying uncertainty, like Guesstimate and Squiggle?
We hope that these ideas will lay the groundwork for an increasingly rigorous system for maximizing our impact on global well-being. We’ve focused on long-term FOs as our primary test case here, but we believe philanthropists and charity evaluators could apply a similar ratings system in many other high-uncertainty contexts.
If you want to share feedback about our methodology, you can reach out to us at research@founderspledge.com.
Notes
-
Another option we’ve considered is the possibility of using probabilistic ratings, e.g., an 80% chance of Charity A winning over Charity B, with a 20% chance of Charity B over Charity A. ↩
-
We use the concept of impact multipliers in the contexts of our general methodology, nuclear philanthropy, and climate philanthropy, among others. ↩
-
The k-factor in chess varies based on the strength of each player. The US Chess Federation uses k=32 for players rated below 2100, k=24 for players rated between 2100–2400, and k=16 for players rated above 2400 to reduce instability amongst the world’s top-rated players. ↩
-
As we do with any new methodology, we’re still iterating on this calculation system and continuously looking for ways to improve it. ↩
-
Bayesian inference gives us an efficient way to adjust beliefs based on new evidence. Each FO’s existing Elo rating informs our prior beliefs, which we can update after a new matchup based on the probability of the matchup’s outcome. A low-rated FO winning over a highly-rated FO is a low-probability event, so it has a greater effect on how much we update our beliefs than a highly-rated FO beating a low-rated FO. See this page for more on Bayesian inference. ↩
-
This system is named after Stanislav Petrov, who is widely credited with having prevented a large-scale nuclear war in September 1983, when he refused to launch a retaliatory nuclear strike on the U.S. after a false alarm in the Soviet satellite warning system. ↩